Depth-Centric Dehazing and Depth-Estimation from
Real-World Hazy Driving Video
AAAI 2025
- Junkai Fan1
- Kun Wang1
- Zhiqiang Yan1
- Xiang chen1
- Shangbin Gao2
- Jun Li*1
- Jian Yang*1
-
1Nanjing University of Science and Technology
2Huaiyin Institute of Technology
Video Results
Abstract
In this paper, we study a challenging problem of simultaneously removing haze and estimating depth from real monocular hazy videos. These tasks are inherently complementary:enhanced depth estimation improves dehazing via the atmospheric scattering model (ASM), while superior dehazing contributes to more accurate depth estimation through the brightness consistency constraint (BCC). To tackle these tasks, we propose a novel depth-centric learning framework that integrates the ASM model with the BCC constraint. Our key idea is that both ASM and BCC rely on a shared depth estimation network. This network simultaneously leverages adjacent dehazed frames to enhance depth estimation using BCC and employs the refined depth cues to more effectively remove haze using ASM. Additionally, we leverage a non-aligned clear video and its estimated depth to independently regularize the dehazing and depth estimation networks. This is achieved by designing two discriminator networks: DMFIR, which enhances high-frequency details in dehazed videos, and MDR, which reduces the occurrence of black holes in low-texture regions. Extensive experiments demonstrate that the proposed method outperforms current state-of-the-art techniques in both video dehazing and depth estimation tasks, especially in real-world hazy scenes.
Motivation
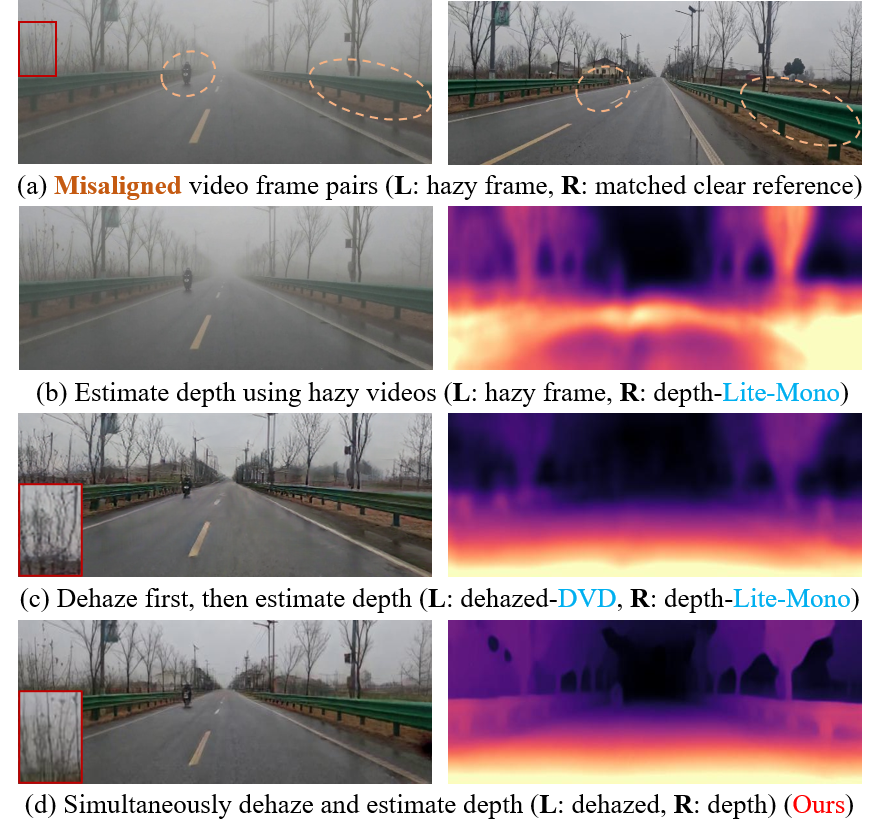
Compare three different approaches to selfsupervised monocular depth estimation in real hazy scenes.
(a) Non-aligned hazy/clear image pairs obtained through the matching algorithm.
(b) Directly estimating depth from hazy video.
(c) Dehaze first, then estimate depth.
(d) Simultaneously dehaze and estimate depth.
Method
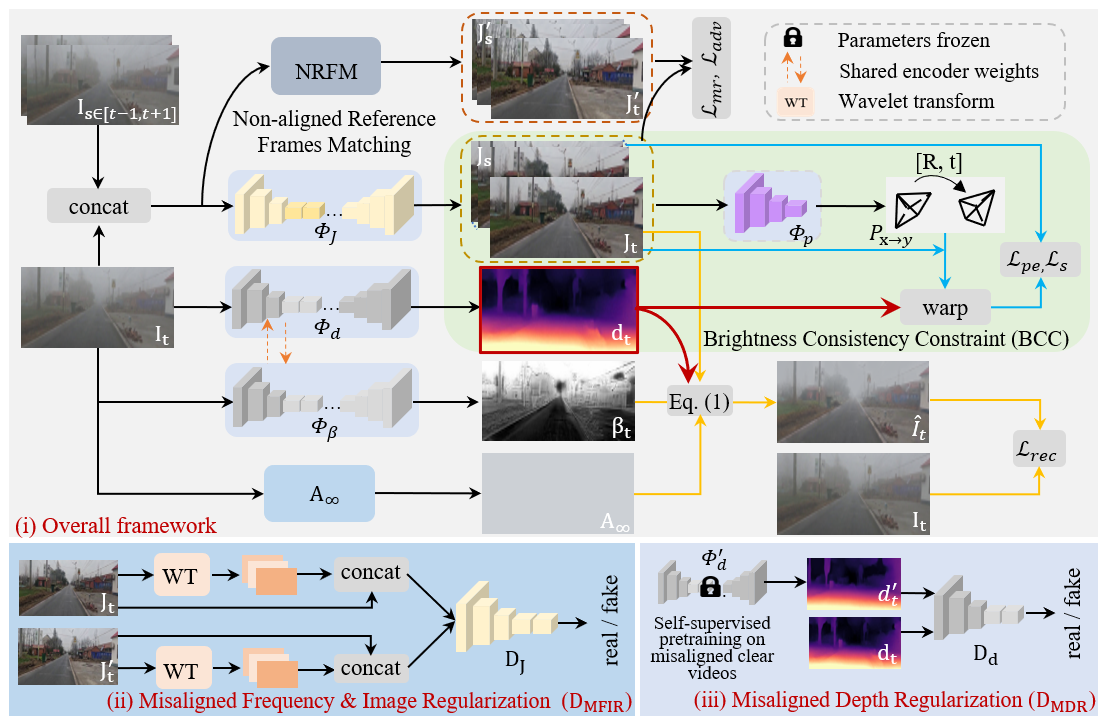
The pipeline of our Depth-Centric Learning (DCL) framework that effectively integrates the atmospheric scattering
model with the brightness consistency constraint through shared depth prediction. DMFIR enhances high-frequency
detail recovery in dehazed frames, while DMDR reduces black holes in depth maps caused by weakly textured regions.
Results
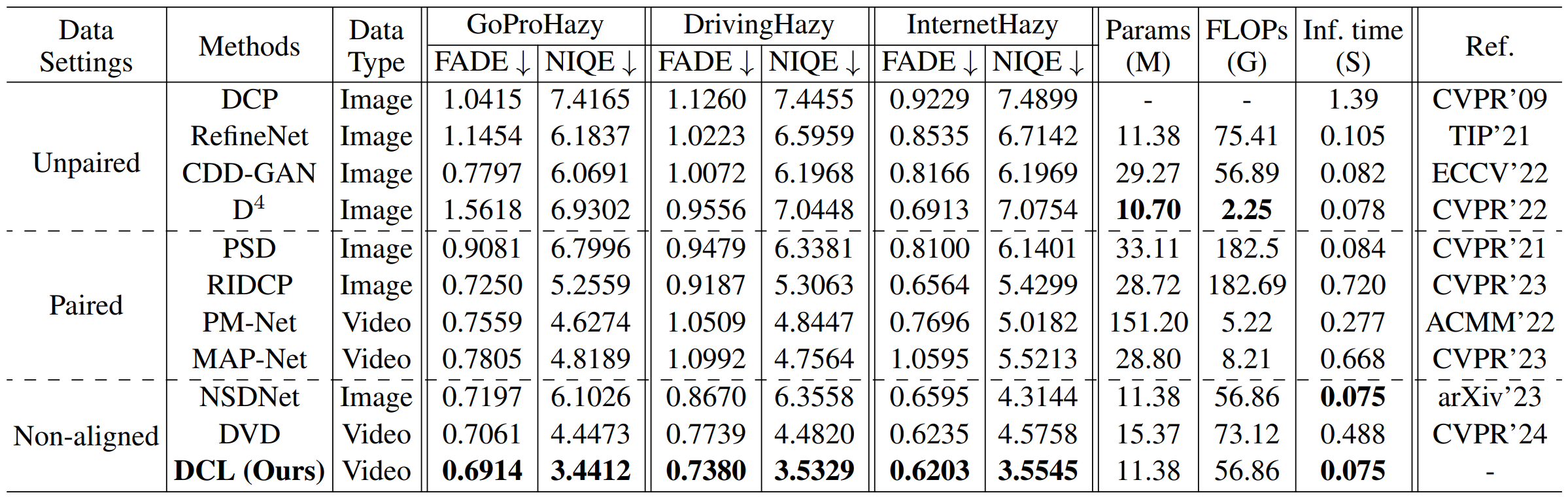
Quantitative results on three real-world hazy video datasets. ↓ denotes the lower the better. For dehazing methods thatrely on ground truth for training, we use Lmr to train them on the GoProHazy dataset. DrivingHazy and InternetHazy were tested with dehazing models trained on GoProHazy. Note that all quantitative results were evaluated at an output resolution of 640×192.
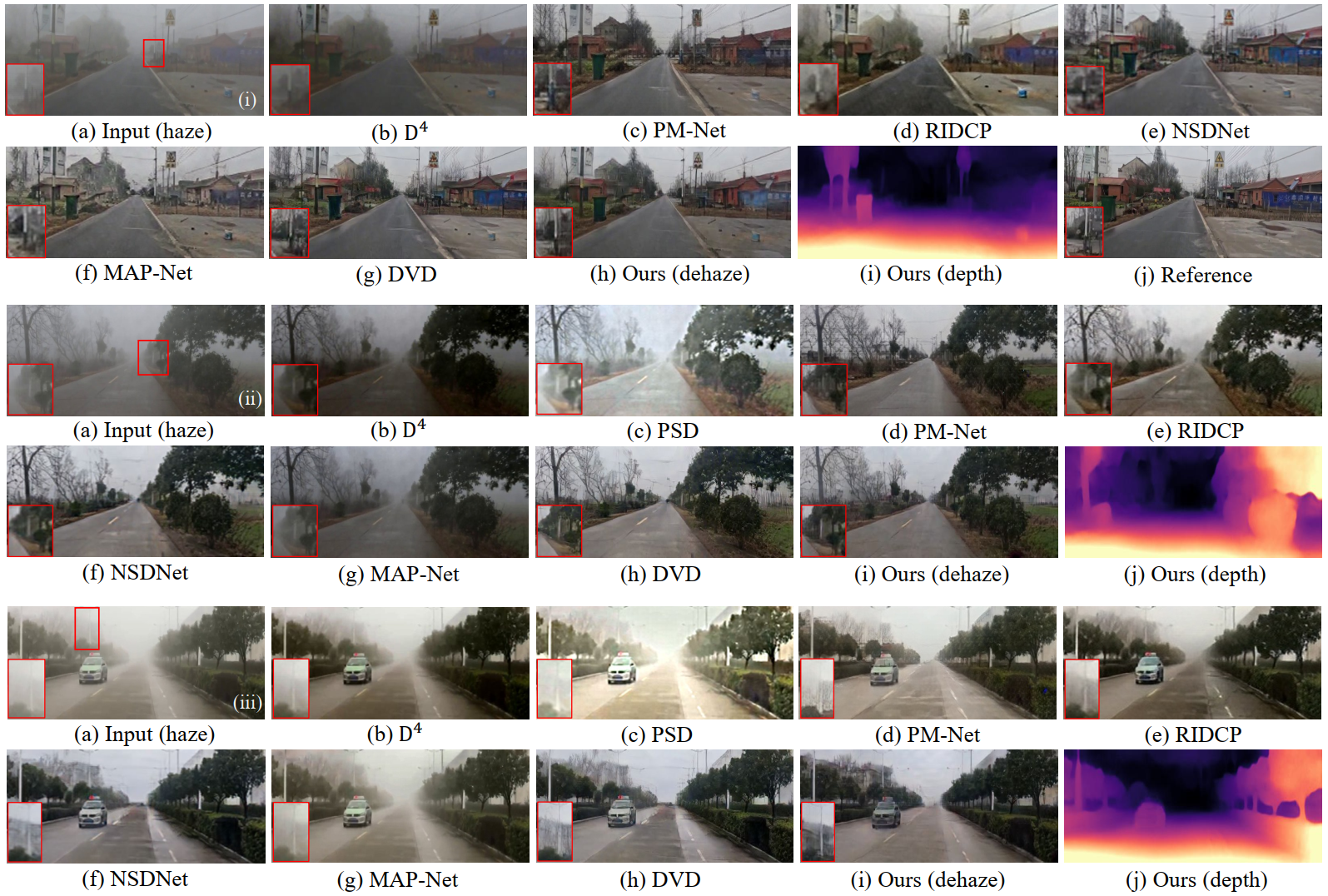
Comparing video dehazing results on GoProHazy (i), DrivingHazy (ii), and InternetHazy (iii), respectively, our method effectively removes disent haze and estimates depth. The red box corresponds to the zoomed-in patch for better comparison.

Quantitative results. We compare our framework with previous state-of-the-art methods on DENSE-Fog dataset. All methods were trained on the GoProHazy dataset. Note that RobustDepth is trained on clear reference videos from GoProHazy, as it uses its own synthetic haze for training.
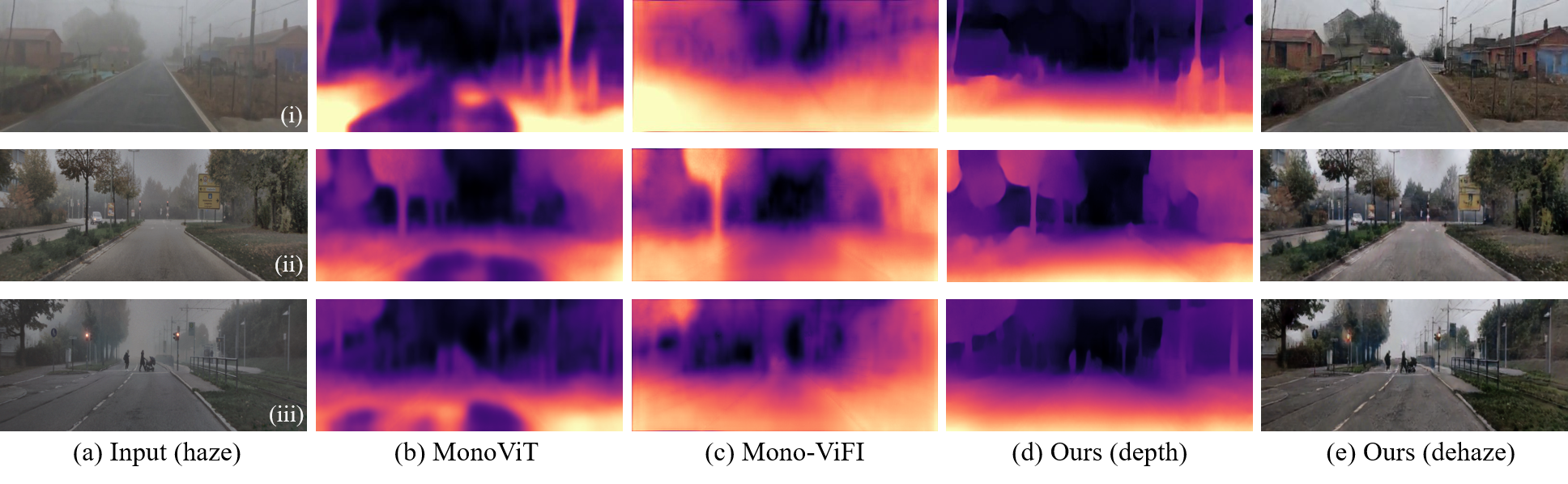
Qualitative results on GoProHazy (i) and DENSE-Fog (ii-dense, iii-light). Our method demonstrates good dehazing generalization and more accurate depth in real hazy scenes.
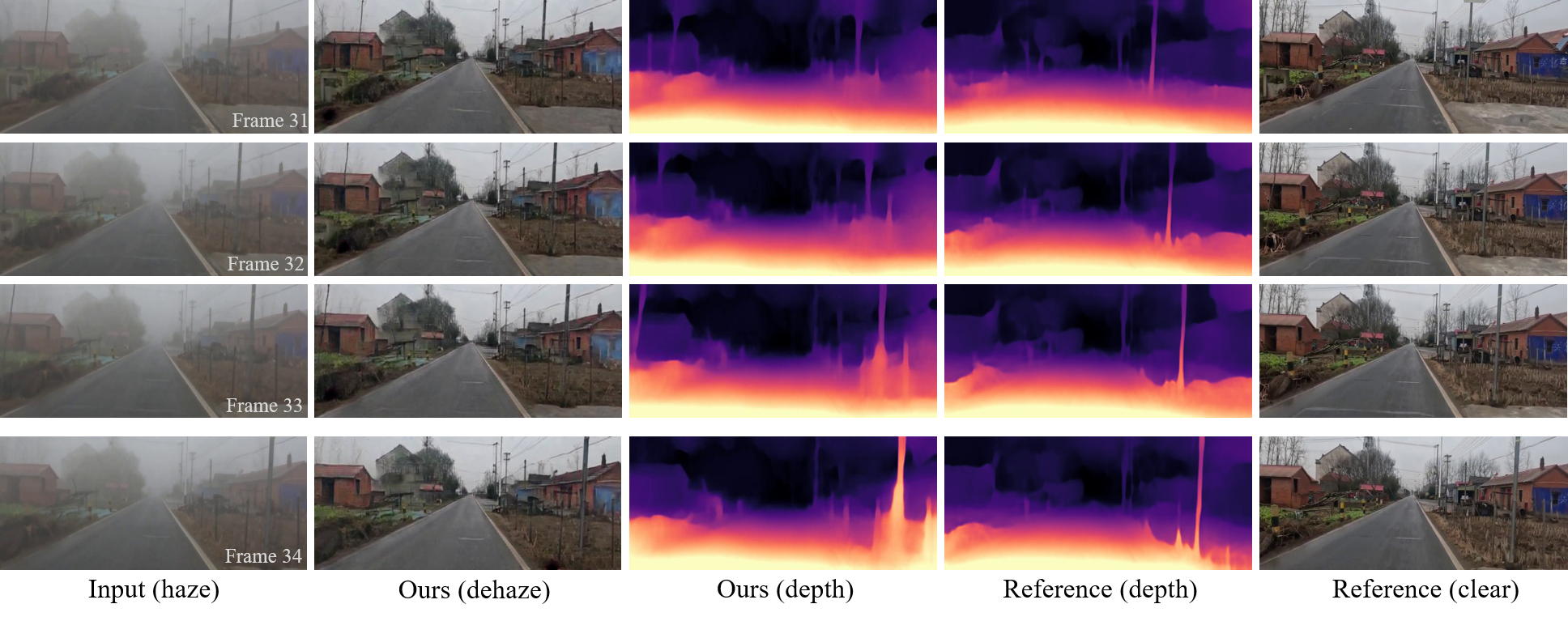
Our method visualizes consecutive frame dehazing and depth estimation results on GoProHazy
Acknowledgements
Thanks to Ricardo Martin-Brualla and David Salesin for their comments on the text, and to George Drettakis and Georgios Kopanas for graciously assisting us with our baseline evaluation.
The website template was borrowed from Michaël Gharbi.